A new machine-learning approach might direct scientists to the most potential extraterrestrial habitats for life.
A recently developed machine-learning instrument might aid in the search for indications of life on Mars and other distant worlds.
Due to the difficulty of collecting samples from other planets, scientists must rely on remote sensing techniques to search for evidence of extraterrestrial life. Hence, any strategy that may assist in directing or refining this search would be quite valuable.
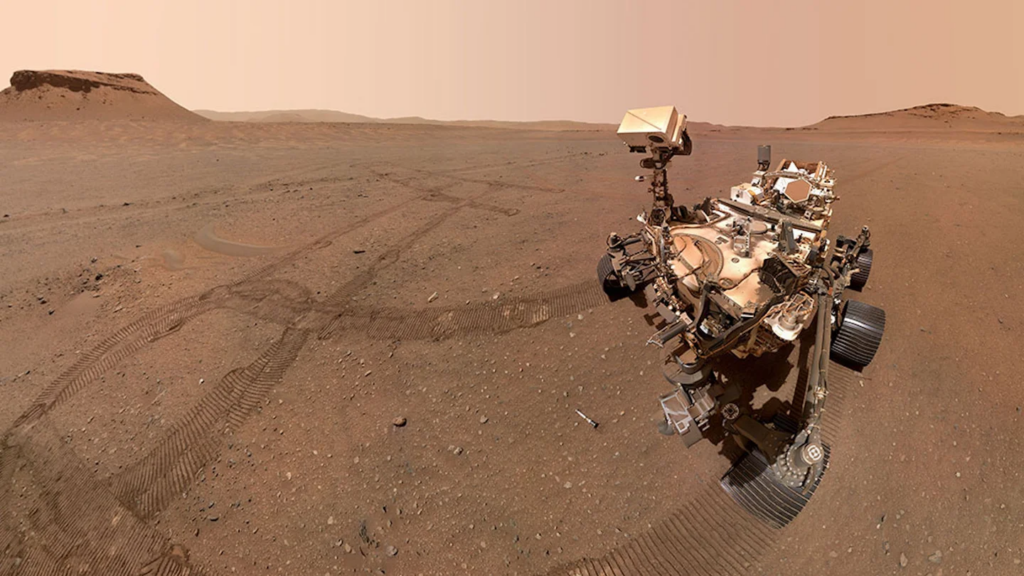
A salt flat at the border of the Chilean Atacama Desert and Altiplano, or high plateau
In light of this, a multidisciplinary team of scientists led by Kim Warren-Rhodes of the SETI (Search for Extraterrestrial Intelligence) Institute in California mapped the sparse lifeforms that inhabit salt domes, rocks, and crystals in the Salar de Pajonales, a salt flat at the border of the Chilean Atacama Desert and Altiplano, or high plateau.
Warren-Rhodes then collaborated with Michael Phillips from the Applied Physics Laboratory at Johns Hopkins University and Freddie Kalaitzis from the University of Oxford to train a machine learning model to recognize the patterns and rules associated with the distribution of life across the harsh region.
This training enabled the model to recognize the same patterns and principles across a vast array of landscapes, including those that may exist on distant worlds.
Combining statistical ecology and artificial intelligence, the researchers showed that their system could locate and identify biosignatures up to 87.5% of the time. This is in comparison to the success rate of random searches, which seldom exceeds 10%.
In addition, the method might reduce the area required for a search by as much as 97%, aiding scientists in their quest for probable biosignatures or chemical evidence of life.
“Our approach enables us to combine the power of statistical ecology and machine learning to identify and forecast the patterns and principles by which wildlife survives and disperses in the hardest places on Earth,” Warren-Rhodes said in a statement (opens in new tab).
We expect that additional astrobiology teams will use our methodology for mapping more habitable environments and biosignatures.
According to the researchers, such machine learning capabilities might be used to robotic planetary missions like that of NASA’s Perseverance rover, which is now searching for signs of life on the bottom of Jezero Crater on Mars.
“Using these models, we can create custom-tailored roadmaps and algorithms to steer rovers to locations with the highest possibility of harboring past or current life,” Warren-Rhodes stated.
Choosing an equivalent for Mars on Earth
The researchers picked Salar de Pajonales as a testing ground for their machine learning model because it is comparable to the desolate and arid terrain of contemporary Mars. The area is a high-altitude, ultraviolet-radiation-blasted salt lakebed at high altitude. Although being regarded extremely unfriendly to life, Salar de Pajonales is home to several organisms.
The scientists gathered over 8,000 photographs and over 1,000 samples from Salar de Pajonales in order to identify photosynthetic bacteria inhabiting the salt domes, rocks, and alabaster crystals of the region. The pigments that these bacteria release constitute a potential biosignature on NASA’s “ladder of life detection,”(opens in a new tab) which is meant to assist scientists in their search for extraterrestrial life within the restrictions of robotic space missions.
The scientists also analyzed Salar de Pajonales using drone footage comparable to photographs of Martian landscape taken by NASA’s Mars Reconnaissance Orbiter’s High-Resolution Imaging Experiment (HIRISE) sensor. This information allowed them to conclude that microbial life in Salar de Pajonales is not dispersed randomly, but rather is concentrated in biological hotspots that are highly correlated with the availability of water.
Machine learning identifies eight possible technosignatures
Difficult extraterrestrial worlds are simpler to locate when people and robots work together.
The team of Warren-Rhodes then trained convolutional neural networks (CNNs) to detect and forecast significant geological characteristics at Salar de Pajonales. Several of these characteristics, including patterned ground and polygonal networks, are also present on Mars. Moreover, the CNN was trained to detect and forecast microhabitats that are most likely to contain biosignatures.
The researchers will continue to train their AI in Salar de Pajonales for the time being, with the intention of testing the CNN’s capacity to forecast the location and distribution of ancient stromatolite fossils and salt-tolerant microbes. This should help it determine if the principles it employs in this search may also be used to the quest for biosignatures in other natural systems with comparable characteristics.
After this, the team intends to continue mapping hot springs, permafrost-covered soils, and rocks in arid valleys, with the objective of training the AI to zero in on suitable homes in other extreme situations on Earth before perhaps investigating those on other worlds.